Maximizing Business Insights: The Role of Data Modeling in Analysis
Understanding data modeling is crucial for effective analysis and decision-making in today's fast-paced business environment. Integrating frameworks like BABOK into a structured curriculum can empower teams to enhance their data management practices, leading to sharper business intelligence insights. Tools such as NumPy facilitate sophisticated data analysis, enabling organizations to harness their data more effectively. Mastering data modeling opens new avenues for predictive analytics and drives better strategic outcomes. As businesses evolve, the role of data modeling becomes increasingly significant. Keep reading to uncover the essential aspects of data modeling and how it can transform the analytical landscape of your organization.
Key Takeaways
- data modeling is essential for effective analysis and informed decision-making
- conceptual, logical, and physical models each serve distinct purposes in data organization
- integrating advanced technologies enhances the capabilities of data models for predictive insights
- regular review and maintenance of models are vital for ensuring data relevance and integrity
- a comprehensive training program equips analysts with the necessary skills for effective data modeling
Understanding the Basics of Data Modeling for Analysis
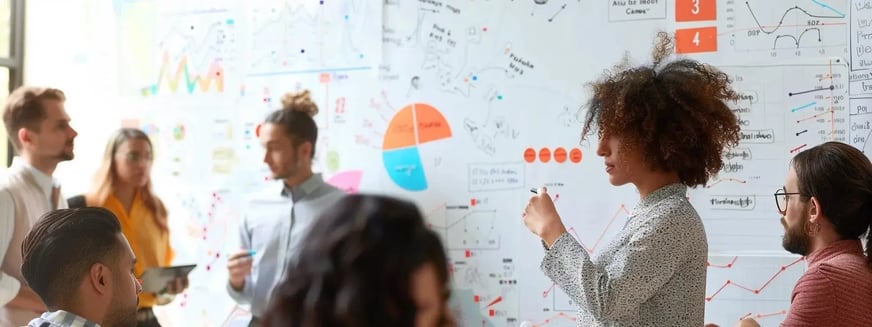
Data modeling serves as the foundation for effective analysis, allowing professionals to structure and visualize information meaningfully. It defines how data is organized, stored, and accessed, which is crucial for informed decision-making. Key components of a data model, including entities, attributes, and relationships, play a significant role in capturing the necessary details to support analysis. Understanding the connection between data modeling and data analysis reveals how structured data can lead to actionable insights. There are various types of data models—conceptual, logical, and physical—each offering a distinct perspective that aids consultants in effectively aggregating data. These models can incorporate conditional logic and facilitate subtyping, allowing for more nuanced interpretations of complex datasets. The application of data modeling principles aligns with best practices outlined in the BABoK.
Defining Data Modeling and Its Importance
Data modeling serves as a critical element in business analysis, providing a structured framework that enhances understanding and interpretation of data. By establishing a hierarchy and defining the level of detail within datasets, professionals can engage in problem-solving with greater creativity. Tools such as Microsoft Power BI leverage these models to visualize data effectively, enabling better communication of insights and fostering informed decision-making in various business contexts.
Key Components of a Data Model
Understanding the key components of a data model is paramount for effective analysis. This framework encompasses entities, attributes, and relationships, enhancing data quality and providing a solid foundation for predictive analytics. Organizations can leverage structured data to drive insights and inform decision-making processes by integrating these elements within a strategic policy or theoretical approach.
The Relationship Between Data Modeling and Data Analysis
The relationship between data modeling and data analysis highlights the importance of structuring data to enhance clarity and usability. Utilizing a relational database allows for efficient sorting of information and facilitates deeper insights during business analysis, as it enables analysts to focus on relevant data points that can drive decisions and strategies. Properly designed data models streamline operations and ensure that vital business elements receive the attention necessary for optimal performance.
- Data modeling provides a structured approach to organizing data.
- Relational databases enhance the sorting capabilities of data sets.
- Effective data models support in-depth business analysis.
- Attention to detail within models leads to better decision-making.
Types of Data Models: Conceptual, Logical, and Physical
Conceptual, logical, and physical data models are essential tools for business analysts aiming to maximize insights from datasets. The lecture on these models often emphasizes their correlation with customer experience, illustrating how each type serves a distinct purpose in organizing data for analysis. By effectively structuring data through these models, analysts can facilitate the export of valuable insights that enhance the overall customer experience and inform strategic decisions.
In crafting data models, the conceptual stage focuses on high-level entities and relationships, laying the groundwork for logical models that delve into the specifics of data attributes and formats. This hierarchy highlights the importance of clarity, helping business analysts streamline processes and illustrate the connection between data points. Analysts advance to the physical model and define how data will be stored and accessed, ensuring efficiency in extracting insights relevant to customer experience management.
Data modeling serves as the backbone for analytical insights, paving the way for more informed predictions. By harnessing structured data, businesses can unlock powerful forecasting capabilities that drive strategic decisions.
How Data Modeling Enhances Predictive Analytics
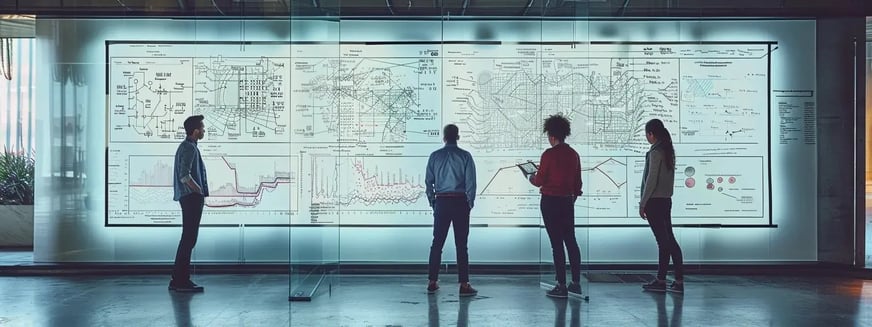
Data modeling plays a pivotal role in enhancing predictive analytics, as it provides the necessary structure for interpreting large datasets. Organizations can build models that refine their approach to business predictions by focusing on data modeling essentials, ensuring a comprehensive understanding of the various dimensions involved. The integration of DevOps principles into data modeling practices fosters efficiency and adaptability, accelerating the process modeling phase. Through practical case studies, businesses have demonstrated how effective data models yielded successful predictive analytics strategies, leading to improved search results and actionable insights that drive performance and growth.
The Role of Data Modeling in Predictive Analysis
Data modeling serves an integral function in predictive analysis across various industries, including health care. Architects of data systems construct robust databases that capture vital information, allowing for accurate forecasting of health trends and patient outcomes. Analysts can effectively inform online and offline healthcare services strategies by identifying deviations in data patterns.
- Data modeling shapes the construction of databases for accurate analysis.
- Healthcare professionals leverage models to predict patient outcomes.
- Deviation analysis helps identify patterns that inform strategies.
- Models support both online and offline analytical processes.
Building Models for Better Business Predictions
Building effective models for better business predictions involves implementing dimensional modeling techniques that enhance the clarity and usability of data. By adopting a logical schema, organizations can facilitate seamless data transformation, enabling data scientists to confidently extract meaningful insights. This structured approach not only improves predictive accuracy but also assists in aligning business strategies with anticipated market trends.
Case Studies: Success Stories of Predictive Analytics
One notable case study in predictive analytics involved a retail company leveraging data visualization techniques to enhance sales forecasting. By utilizing descriptive statistics, they identified patterns in customer purchasing behavior, linking specific time periods with higher sales volumes. The results of this analysis enabled the company to optimize inventory management at a granular level, ensuring that each cell in its supply chain was adequately stocked based on predictive insights.
A healthcare organization implemented predictive analytics to improve patient outcomes by analyzing historical data and employing a clear concept of risk factors. Their analysis utilized descriptive statistics to uncover critical patterns in patient demographics and treatment responses, allowing healthcare providers to tailor interventions effectively. Data visualization tools were then used to present these insights, enabling stakeholders to make informed decisions quickly.
A prominent firm adopted predictive analytics to enhance product development cycles in the technology sector. The company gained insights into feature usage and customer preferences by applying descriptive statistics to user engagement metrics. This analysis, complemented by sophisticated data visualization techniques, provided critical feedback for their development teams to refine future iterations of their products, driving both user satisfaction and profitability.
Building on the power of predictive analytics, the right tools can elevate data modeling to new heights. Discover the essential software options that can streamline this vital process and drive impactful results.
Essential Tools and Software for Effective Data Modeling
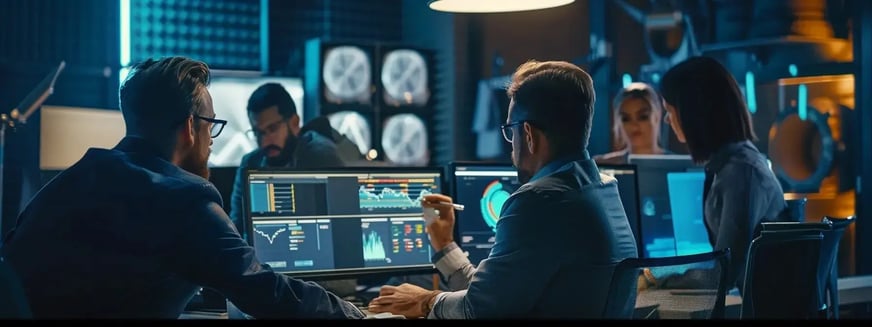
Equipping businesses with the necessary tools to streamline data modeling is vital for extracting optimal insights. A variety of leading data modeling programs are available, each offering unique functionalities that cater to different organizational requirements. Professionals must consider factors such as the ability to create detailed conceptual models and generate insightful charts when selecting the right engine for their needs. Moreover, integrating data modeling software with existing systems ensures seamless workflows and maximizes operational efficiency. Understanding these elements empowers businesses to derive significant value from their data, ultimately supporting informed decision-making processes.
Overview of Leading Data Modeling Tools
Leading data modeling tools play a crucial role in enhancing data architecture and providing businesses with the necessary resources for effective analysis. Tools such as MySQL enable professionals to manage raw data efficiently, allowing for the extraction of meaningful metrics, including the median. These resources streamline the modeling process, facilitating a more accurate understanding of complex datasets and supporting informed decision-making.
Selecting the Right Tool for Your Business Needs
Choosing the appropriate data modeling tool significantly impacts the effectiveness of business processes across various sectors. Organizations benefit from platforms that facilitate data science applications and enhance visualization capabilities, especially in healthcare. Selecting a tool that effectively analyzes variance in datasets is crucial for deriving actionable insights that drive performance.
- Assess organizational needs to identify key features necessary for data modeling.
- Evaluate tools that support healthcare analytics and data science
- Prioritize visualization capabilities for a better understanding of datasets.
- Consider tools that provide insights into variance for informed decision-making.
Integrating Data Modeling Software With Existing Systems
Integrating data modeling software with existing systems enhances business analytics by streamlining the workflow across various departments. A well-structured data warehouse enables teams to utilize advanced techniques such as linear regression and data mining, which amplify the potential of analytical insights. By ensuring compatibility between systems, organizations can achieve a cohesive environment where data flows seamlessly, facilitating informed decision-making and driving performance improvements.
With the right tools at hand, data professionals can craft robust models that form the backbone of insightful analysis. Now, let’s turn our attention to techniques that sharpen these models, maximizing their effectiveness for data-driven decision-making.
Techniques to Optimize Your Data Models for Analysis
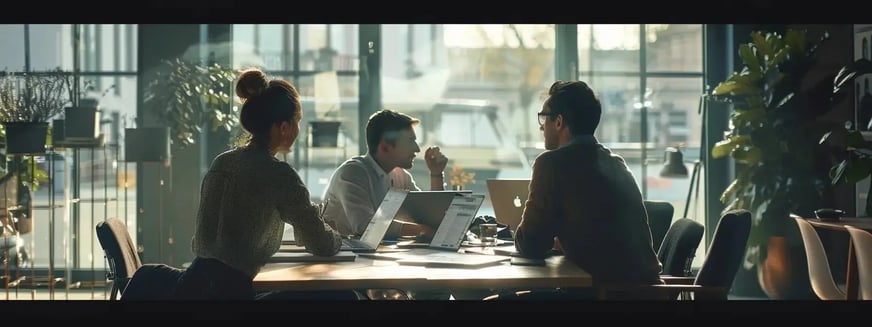
To enhance user experience through effective data analysis, mastering optimization techniques in data model design is essential. Implementing best practices allows organizations to construct clear diagrams that accurately represent data relationships and flows. By being mindful of common pitfalls during the modeling process, teams can prevent potential issues that may undermine analysis accuracy and efficiency. Furthermore, regular review and maintenance of data models ensure they remain relevant and aligned with evolving business requirements, ultimately supporting the employment of robust analytics capabilities. These measures collectively improve the quality of insights derived from data, driving informed decision-making across various sectors.
Best Practices in Data Model Design
Best practices in data model design focus on ensuring clarity and effectiveness for analytical purposes. Professionals should prioritize readability and logical structure to facilitate an intuitive understanding of data relationships. By incorporating statistical functions and visualization techniques such as box plots, analysts can better interpret data distributions and outliers, ultimately enhancing the quality of insights derived from the models:
- Emphasize readability and logical clarity in model design.
- Utilize statistical functions to derive actionable insights.
- Incorporate box plots to visualize data distributions.
- Regularly review and update models to maintain relevance.
Avoiding Common Data Modeling Pitfalls
Avoiding common pitfalls in data modeling requires careful consideration of design choices and understanding how data interacts within systems. Experts recommend prioritizing the selection of primary keys as they uniquely identify records and prevent redundancy, thus enhancing data integrity. Using an advanced programming language facilitates the implementation of these strategies, allowing for a versatile palette of techniques to construct effective models.
- Prioritize the selection of primary keys for data integrity.
- Utilize advanced programming languages for efficient model design.
- Implement expert recommendations for avoiding redundancy in data.
Regular Review and Maintenance of Data Models
Regular review and maintenance of data models ensure they remain aligned with dynamic business needs and compliance standards, including data governance regulations. Attention to foreign key relationships is vital, as they help maintain data integrity by linking related records effectively. Software engineering principles guide this process, allowing teams to implement changes systematically during training seminars or preparation for exams focused on data management best practices.
Activity | Purpose | Frequency |
---|---|---|
Review Data Models | Ensure alignment with business needs | Quarterly |
Update Foreign Keys | Maintain data integrity | As needed |
Implement Changes | Adapt to new requirements | Ongoing |
Conduct Training Seminars | Educate teams on best practices | Annually |
Mastering data modeling techniques sets the foundation for powerful insights. Now, it’s time to equip your team with essential skills to harness that potential fully.
Training Your Team in Data Modeling Essentials
Equipping analysts with the necessary skills in data modeling is imperative for maximizing business insights. Understanding the intricacies of systems and cloud computing can significantly enhance their ability to construct a robust logical data model. Developing a comprehensive training program that focuses on key competencies is essential, allowing analysts to navigate complex data structures efficiently. This program should incorporate practical applications of window functions and explore various resources and courses for further learning. By fostering a culture of continuous improvement, organizations can ensure their teams are well-versed in data modeling essentials, ultimately leading to more effective analysis and informed decision-making.
Key Skills for Data Modelers in Business Analysis
Data modelers in business analysis must cultivate a blend of technical and creative skills to ensure effective communication and innovation. Mastery of data architecture enables them to design robust frameworks that support analytical processes, while proficiency in storytelling allows them to present complex findings in a relatable manner. Additionally, understanding machine learning principles enhances their ability to leverage advanced algorithms, thereby driving deeper insights in accordance with organizational terms of service.
Developing a Training Program for Your Analysts
Developing a comprehensive training program for analysts in data modeling is essential for enhancing business intelligence capabilities. This program should emphasize the use of tools such as Power Query and techniques like scatter plots to help analysts visualize data relationships effectively. By incorporating lessons on syntax and practical applications, professionals can cultivate a skill set that enables them to derive actionable insights from complex datasets.
Resources and Courses for Further Learning
Organizations looking to enhance their data modeling capabilities can benefit from various resources and courses tailored for data analytics. These educational offerings often cover critical concepts such as the star schema, which simplifies the organization of data structures, making it easier for analysts to query and report on categorical variables. By mastering these topics, professionals gain the necessary skills to formulate hypotheses and extract valuable insights from datasets, ultimately bolstering the organization's analytical proficiency.
As organizations increasingly rely on data-driven decisions, the landscape of data modeling evolves rapidly. Anticipating what lies ahead in this dynamic field will empower business analysts to stay ahead of the curve.
The Future of Data Modeling in Business Analysis
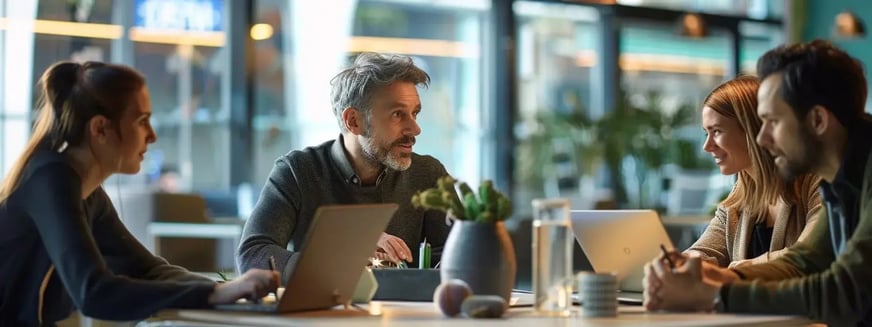
The evolving landscape of enterprise data requires organizations to adapt their modeling strategies to capitalize on emerging trends that enhance analytical capabilities. The integration of machine learning is particularly noteworthy, as it empowers analysts to mine vast datasets, providing predictive insights that align with changing business dynamics. Additionally, the shift towards utilizing surrogate keys simplifies the structure of data relationships, optimizing performance and accelerating query responses. Understanding the normal distribution of data helps businesses identify patterns and outliers effectively, thereby informing strategic decisions. To maintain a competitive edge, organizations must prepare for future innovations in data modeling that leverage advanced analytics and artificial intelligence, ultimately driving increased efficiency and insight generation.
Emerging Trends in Data Modeling and Analysis
Emerging trends in data modeling emphasize the importance of incorporating advanced parameters that enhance database design for more efficient data management. Analysts are increasingly utilizing histograms within Microsoft Excel as a method for visualizing data distributions, aiding in the identification of potential outliers that may skew analytical results. This approach enhances the accuracy of data interpretations and supports strategic decision-making processes by providing clearer insights into complex datasets.
How AI and Machine Learning Are Shaping Data Modeling
Artificial intelligence (AI) and machine learning are significantly transforming the landscape of data modeling by enhancing the interface between analysts and data, facilitating a more effective analysis of customer behaviors and preferences. These technologies allow organizations to model complex variables and assess probabilities associated with various outcomes, leading to improved customer insights and more accurate forecasting capabilities. As businesses leverage AI-driven models, they gain a deeper understanding of customer interactions, ultimately driving informed decision-making and strategic planning.
Preparing Your Business for Future Data Modeling Innovations
Organizations must foster a versatile workspace that embraces emerging technologies to effectively prepare for future data modeling innovations. Financial analysts play a critical role in identifying trends and leveraging tools such as Matplotlib to visualize complex datasets. By focusing on enhancing data aggregation capabilities, businesses will position themselves to adapt swiftly to changing analytical needs.
- Foster a versatile workspace for innovation.
- Engage financial analysts to identify trends.
- Utilize Matplotlib for data visualization.
- Enhance data aggregation capabilities for adaptability.
Conclusion
Data modeling plays a vital role in enhancing business insights by providing a structured framework for organizing and interpreting data. It enables professionals to develop effective predictive analytics strategies, resulting in informed decision-making and improved operational efficiency. Organizations can uncover valuable patterns and insights that drive growth by utilizing various data models and advanced tools. Ultimately, effective data modeling empowers businesses to adapt to changes and optimize performance in a competitive landscape.
You May Also Like
These Related Stories
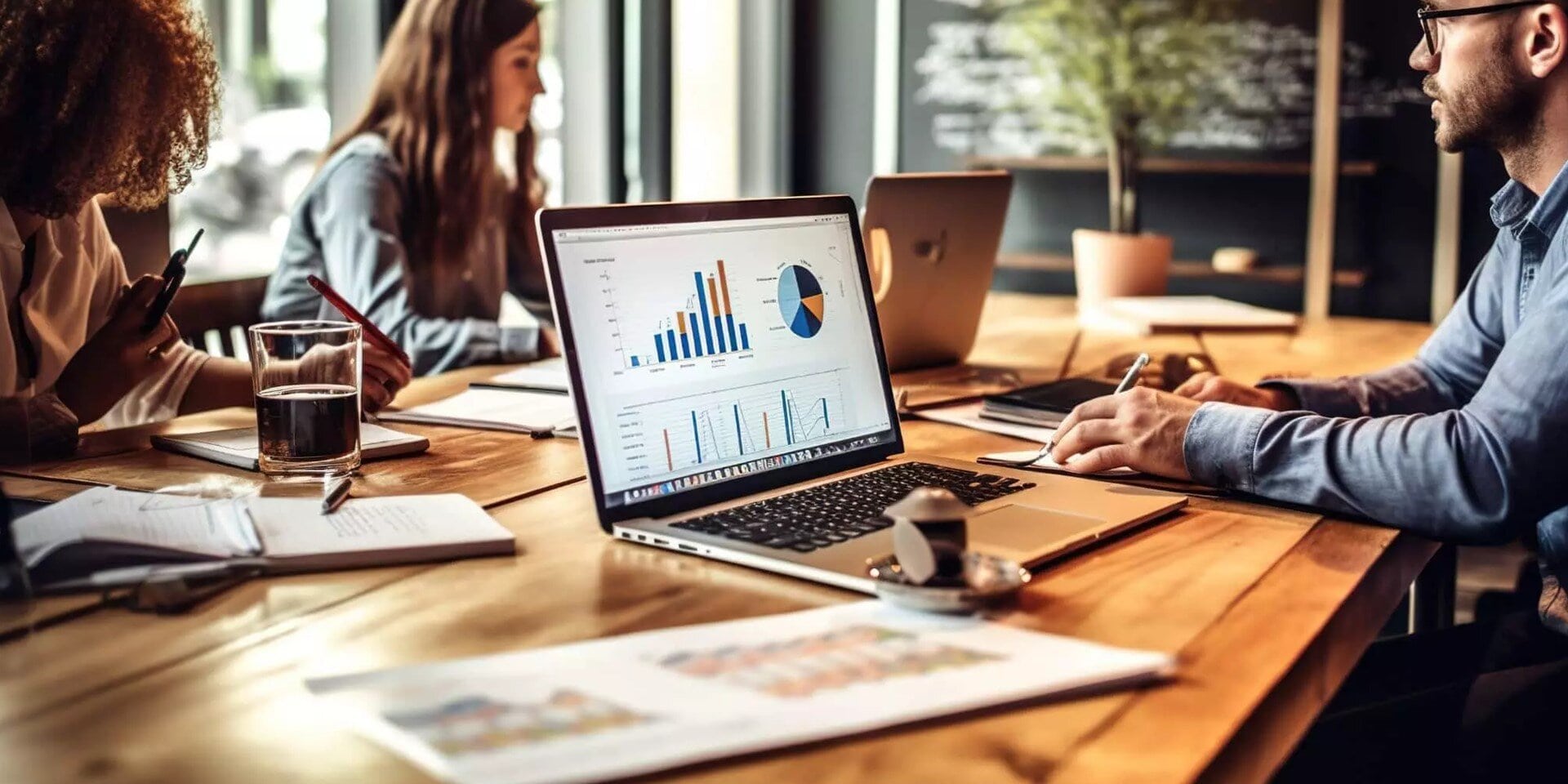
Process Modeling vs. Process analysis | Free eBook
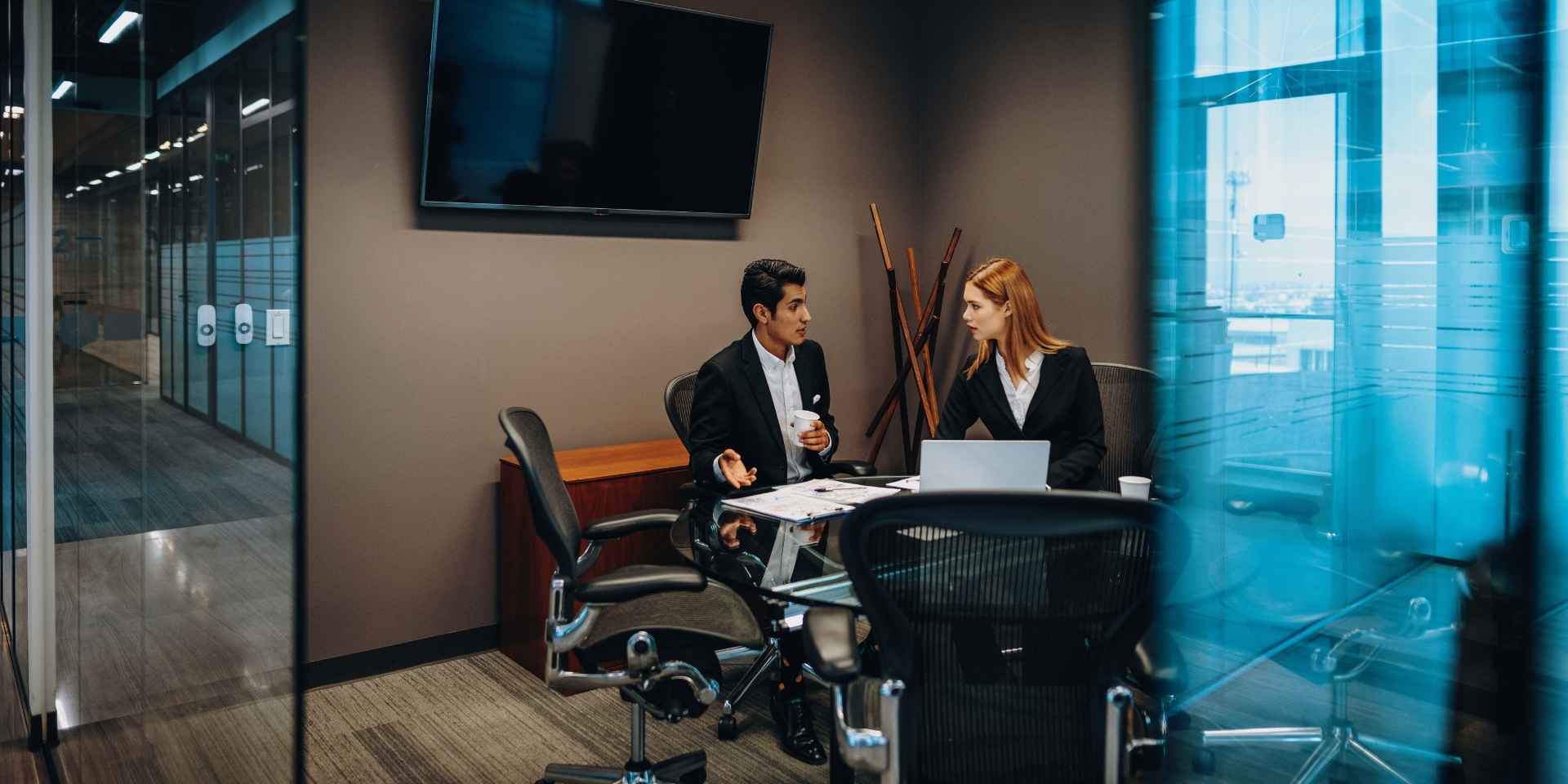
FP&A Modeling: Skills & Techniques
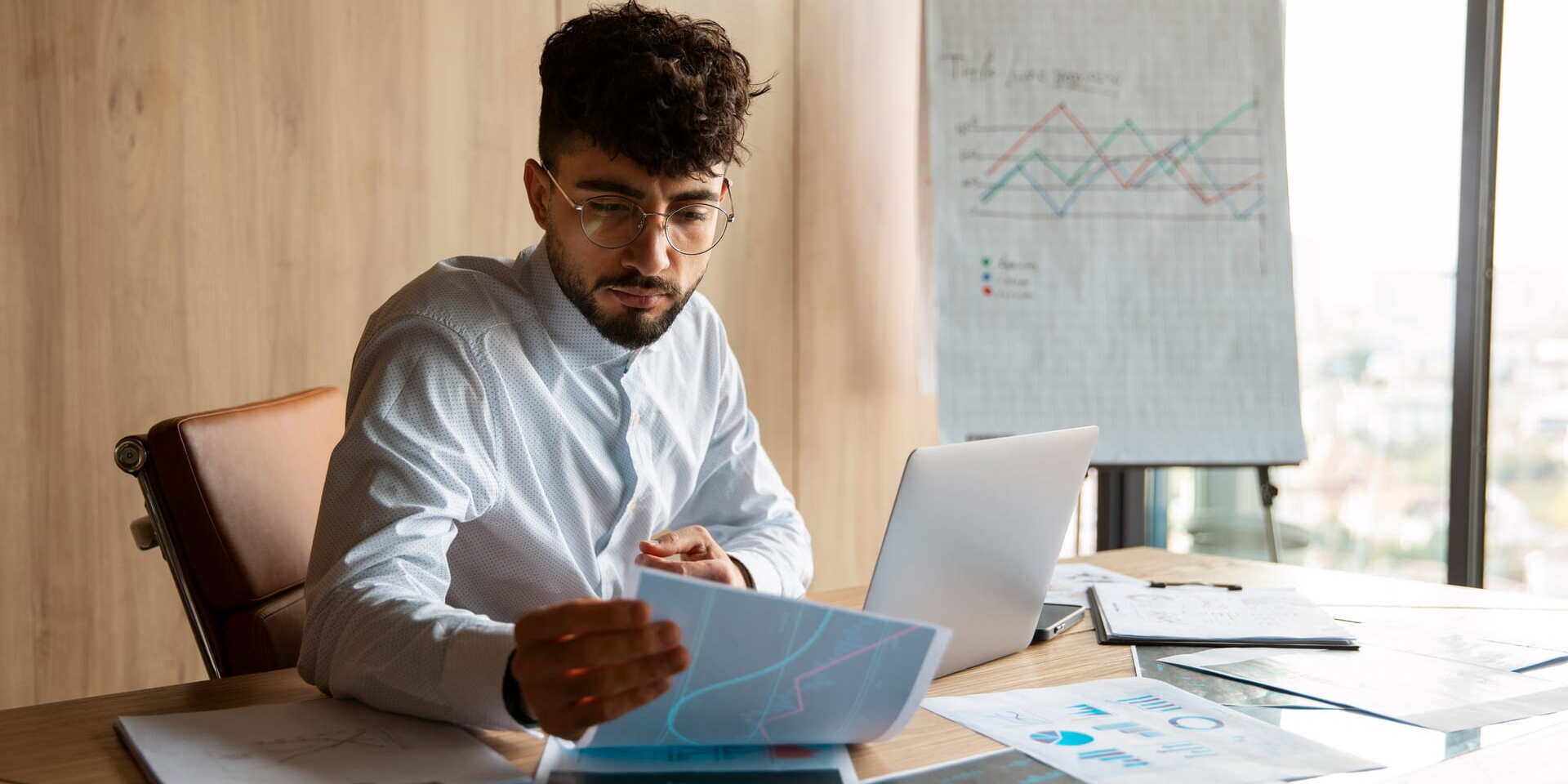
No Comments Yet
Let us know what you think