Data Mining: History, Techniques, Advantages, and Examples
Unearthing hidden treasures, unlocking valuable insights, and paving the way for informed decision-making – this is the power of data mining. In today's digital age, where information is abundant and overwhelming, businesses need a tool to extract meaningful patterns and knowledge from vast datasets. That's where data mining comes into play! It acts as a skilled detective, meticulously sifting through mountains of data to uncover golden nuggets that can revolutionize how businesses operate. Join us on this exciting journey as we delve deep into the world of data mining and explore its fascinating history, key benefits for business analysts, essential steps involved in the process, as well as its limitations. So grab your magnifying glass; it's time to dig into the captivating realm of data mining!
What is Data Mining?
What is data mining, you ask? Well, imagine a vast ocean of information – raw and unstructured. Data mining is the process of diving into this sea of data, exploring its depths to extract valuable insights, patterns, and relationships that may not be immediately apparent. It's like panning for gold in a river; you need patience and skill to separate the valuable nuggets from the debris. Sometimes, data mining may be incorrectly referred by few as database mining as data mined are maintained in databases.
At its core, data mining combines elements from various fields, such as statistics, machine learning, artificial intelligence (AI), and database systems. Applying sophisticated algorithms and techniques to large datasets helps businesses transform raw data into actionable knowledge.
The ultimate goal of data mining is to uncover hidden gems that can drive strategic decision-making. Whether predicting customer behavior patterns or identifying market trends before they emerge – data mining empowers businesses with invaluable foresight.
By leveraging these insights gained through data mining techniques, companies can effectively optimize their marketing strategies by targeting specific customer segments. They can also enhance operational efficiency by identifying bottlenecks or streamlining processes based on patterns identified within their operations.
Data mining is a powerful tool for business analysts to gain a competitive edge in today's fast-paced world. But how exactly does it work? Let's dive deeper into the intricate steps involved in the process!
History of data mining
Data mining, a term that might seem recent and trendy, actually has its roots in the 1960s. It emerged as a concept within the field of artificial intelligence and was initially referred to as "knowledge discovery in databases." The goal was to develop algorithms and techniques that could extract valuable insights from large sets of data.
In the 1970s, researchers began exploring different approaches to data mining. One notable development was the creation of the Apriori algorithm for association rule mining. This algorithm allowed analysts to identify relationships between different variables in a dataset.
As technology advanced in the following decades, so did data mining techniques. In the 1990s, companies started harnessing data mining for business with the rise of powerful computers and storage capabilities. They realized that by analyzing vast amounts of customer information, they could uncover patterns and trends that would give them a competitive edge.
Today, data mining is integral to many industries, such as finance, healthcare, marketing, and more. With advancements in machine learning and artificial intelligence technologies, businesses can now analyze complex datasets faster and more accurately than ever before.
The history of data mining demonstrates how it has evolved from an academic pursuit to becoming a vital tool for businesses looking to gain insights from their data. By understanding this historical context, we can appreciate how far we have come in our ability to extract knowledge from vast amounts of information.
How does data mining help business analysts?
Data mining plays a crucial role in assisting business analysts to make informed decisions and gain valuable insights from large volumes of data. By utilizing advanced algorithms and techniques, data mining helps extract patterns, correlations, and trends that may not be easily noticeable through traditional analysis methods.
One way data mining supports business analysts is by identifying customer behavior patterns. Businesses can understand their customers better by analyzing purchase history, browsing habits, and demographic information. This knowledge enables them to personalize marketing strategies and offer targeted recommendations or promotions.
Furthermore, data mining assists in predicting future trends and behaviors. Pattern recognition and statistical models make it possible to accurately forecast market demand or anticipate consumer preferences. This allows businesses to proactively adjust their strategies accordingly.
Data mining also aids in risk assessment for businesses. By examining historical data on fraudulent activities or financial irregularities, organizations can develop predictive models that detect potential threats before they occur. Consequently, this helps minimize losses and safeguard the company's assets.
Moreover, with the help of data mining techniques such as clustering or association rules discovery, analysts can identify hidden relationships within previously unknown datasets. These discoveries can lead to innovative ideas for product development or process improvement.
In conclusion (as per instructions), incorporating data mining into the analytical process empowers business analysts with deeper insights into customer behavior patterns, better prediction capabilities for future trends, enhanced risk management strategies, and new opportunities for innovation within an organization's operations.
Steps for Data Mining
Data mining is a process that involves extracting valuable insights and patterns from large datasets. It enables businesses to make informed decisions and gain a competitive edge in today's data-driven world. To successfully mine data, analysts follow a series of steps.
- Problem Definition: The first step in data mining is clearly defining the problem or objective that needs to be addressed. This helps narrow the focus of analysis and ensures that the right techniques are applied.
- Data Collection: Once the problem is defined, relevant data must be gathered from various sources such as databases, spreadsheets, or even social media platforms. The quality and quantity of data play a crucial role in obtaining accurate results.
- Data Cleaning: Raw data often contains errors, inconsistencies, or missing values that can affect the accuracy of analysis. In this step, analysts clean and preprocess the data by removing duplicates, handling missing values, and transforming variables as needed.
- Exploratory Data Analysis: Exploring and understanding the dataset through visualizations and descriptive statistics is important before diving into complex algorithms. This helps identify trends, outliers, correlations, or any other interesting patterns within the dataset.
- Model Building: Once familiar with the dataset characteristics, analysts select appropriate modeling techniques based on their objectives - whether it's classification (predicting categories), regression (predicting numerical values), clustering (grouping similar instances), or association rule mining (finding relationships).
- Model Evaluation: After constructing models using machine learning algorithms like decision trees or neural networks, they need to be evaluated for their performance using various metrics such as accuracy, precision, recall, etc.
- Interpretation & Deployment: Lastly, the results obtained from these models need to be interpreted so business stakeholders can understand their implications. Insights gained should drive actionable strategies rather than remain just theoretical concepts. These strategies can then be implemented to improve business operations and maximize outcomes.
Advantages of Data Mining
Data mining offers numerous advantages to businesses and analysts alike. It allows organizations to gain valuable insights from their vast amounts of data. By analyzing this data, patterns, trends, and relationships that may have otherwise gone unnoticed can be uncovered. These insights can then be used to make informed business decisions and drive strategic planning.
Another advantage of data mining is its ability to enhance customer relationship management (CRM). Businesses can tailor their marketing efforts by analyzing customer behavior and preferences. This personalized approach increases customer satisfaction and boosts sales and brand loyalty.
Furthermore, data mining aids in risk assessment and fraud detection. It enables organizations to identify suspicious activities or anomalies within their datasets that may indicate fraudulent behavior. By detecting these irregularities early on, companies can mitigate potential risks and protect themselves against financial losses.
Additionally, data mining helps businesses improve operational efficiency by identifying bottlenecks or inefficiencies in processes. By pinpointing areas for improvement, organizations can streamline operations, reduce costs, and increase productivity.
Data mining contributes to competitive advantage by uncovering market trends and predicting future demand patterns. This allows companies to stay ahead of the competition by adapting their products or services based on consumer needs.
In conclusion, the advantages offered by data mining are undeniable; it provides valuable insights for decision-making purposes while enhancing CRM efforts, detecting fraud, improving operational efficiency, and gaining a competitive edge. Businesses that harness the power of data mining are better equipped to navigate today's complex marketplace successfully. By leveraging the benefits provided by this analytical tool, organizations can unlock hidden opportunities and optimize their overall performance in an ever-evolving business landscape.
Limitations of Data Mining
While data mining offers valuable insights and opportunities for businesses, it also faces certain limitations. Understanding these limitations is crucial in order to make informed decisions based on the results obtained from data mining.
One limitation is the quality of the data. Data mining heavily relies on large datasets, but if the data provided is incomplete or inconsistent, it can lead to inaccurate results. Inaccurate or biased data can skew the outcomes and hinder decision-making processes.
Another limitation lies in privacy concerns. With access to vast amounts of personal information, there are ethical considerations about how this data is used and stored. Protecting customer privacy should be a top priority when conducting any kind of analysis using personal information.
Data mining also requires skilled analysts who possess both technical expertise and domain knowledge. Without such expertise, interpreting the results accurately becomes challenging and may lead to misinterpretation.
Additionally, scalability can pose a limitation for organizations with limited resources. As datasets grow larger, more powerful hardware infrastructure may be required for efficient analysis.
While data mining helps uncover patterns and relationships in historical data, its predictive power is not foolproof. Predictions made based on past trends may not always hold true in future scenarios due to unforeseen events or changes in market conditions.
Understanding these limitations allows businesses to approach data mining with caution while leveraging its benefits effectively.
Data Mining Worked Out Example
Let us learn data mining techniques by means of an example. Governance, Risk, and Compliance (GRC) management system is developed for the ITES and IT domain. The primary goal of the GRC management system is to help organizations implement Governance, Quality, and Information Security Management Systems in an integrated manner. It has various features, one of which is to plan and track projects and programs using standards such as CMMI, ISO 9001, and ISO 27001, etc.
In the following table, defect details with associated characteristics are provided. The aim is to predict the required time for the new defect based on past delivery details.
Application |
Architecture |
Skills |
No of Cis |
Application Familiarity |
Dependency |
Clarification |
CMS |
ASP |
L |
1 |
L |
No |
No |
CMS |
Oracle |
M |
1 |
M |
No |
Yes |
CMS |
Oracle |
M |
1 |
M |
No |
Yes |
CMS |
Oracle |
H |
12 |
L |
Yes |
Yes |
CMS |
ASP |
H |
13 |
M |
Yes |
Yes |
CMS |
ASP |
H |
3 |
M |
No |
No |
CMS |
Oracle |
M |
3 |
L |
No |
Yes |
CMS |
Oracle |
M |
5 |
L |
No |
Yes |
CMS |
ASP |
L |
2 |
L |
No |
No |
CMS |
ASP |
L |
1 |
L |
No |
Yes |
CMS |
ASP |
M |
6 |
M |
No |
Yes |
CMS |
Oracle |
L |
1 |
L |
No |
Yes |
CMS |
ASP |
L |
3 |
L |
No |
Yes |
CMS |
ASP |
L |
1 |
L |
No |
Yes |
CMS |
ASP |
L |
1 |
L |
No |
Yes |
CMS |
ASP |
L |
1 |
M |
No |
Yes |
CMS |
Oracle |
M |
1 |
M |
No |
Yes |
CMS |
Oracle |
M |
1 |
M |
No |
No |
CMS |
Oracle |
L |
1 |
L |
No |
Yes |
CMS |
Oracle |
M |
2 |
M |
No |
No |
GET |
COM |
M |
2 |
L |
No |
Yes |
GET |
COM |
M |
3 |
L |
No |
No |
GET |
COM |
M |
3 |
L |
No |
Yes |
GET |
Oracle |
M |
3 |
M |
Yes |
No |
GET |
Oracle |
L |
4 |
L |
Yes |
No |
GET |
Oracle |
M |
4 |
M |
Yes |
No |
GET |
ASP |
M |
1 |
L |
No |
Yes |
GET |
ASP |
M |
1 |
M |
No |
Yes |
GET |
ASP |
L |
1 |
L |
No |
No |
DBSynch Engine |
VB |
M |
4 |
L |
No |
Yes |
DBSynch Engine |
VB |
M |
4 |
L |
No |
Yes |
GET |
Oracle |
H |
1 |
M |
Yes |
No |
CMS |
ASP |
M |
3 |
M |
Yes |
Yes |
CMS |
ASP |
L |
2 |
L |
No |
No |
CMS |
ASP |
M |
3 |
M |
No |
Yes |
CMS |
Oracle |
L |
2 |
L |
Yes |
No |
CMS |
ASP |
L |
3 |
L |
Yes |
No |
CMS |
Oracle |
H |
2 |
M |
Yes |
Yes |
CMS |
Oracle |
M |
1 |
M |
Yes |
Yes |
CMS |
Oracle |
M |
1 |
M |
No |
Yes |
CMS |
ASP |
M |
3 |
H |
Yes |
No |
CMS |
Oracle |
L |
1 |
L |
Yes |
No |
CMS |
ASP |
H |
1 |
M |
No |
Yes |
ASP |
H |
H |
Yes |
Yes |
||
COM |
M |
M |
No |
No |
||
Oracle |
L |
L |
||||
VB |
||||||
Others |
||||||
Regression model:
Regression Statistics |
||||||||
Multiple R |
0.452268262 |
|||||||
R Square |
0.204546581 |
|||||||
Adjusted R Square |
0.045455897 |
|||||||
Standard Error |
13.00020932 |
|||||||
Observations |
43 |
|||||||
ANOVA |
||||||||
|
df |
SS |
MS |
F |
Significance F |
|||
Regression |
7 |
1521.059515 |
217.2942164 |
1.285723189 |
0.285866481 |
|||
Residual |
35 |
5915.190485 |
169.0054424 |
|||||
Total |
42 |
7436.25 |
|
|
|
|||
|
Coefficients |
Standard Error |
t Stat |
P-value |
Lower 95% |
Upper 95% |
Lower 95.0% |
Upper 95.0% |
Intercept |
13.27606758 |
15.10070223 |
0.879168888 |
0.385306066 |
-17.38002519 |
43.93216035 |
-17.38002519 |
43.93216035 |
Application |
-6.660924277 |
3.96752358 |
-1.678861926 |
0.102086712 |
-14.71543519 |
1.393586639 |
-14.71543519 |
1.393586639 |
Architecture |
5.071853789 |
3.126248855 |
1.622344869 |
0.113704349 |
-1.274776551 |
11.41848413 |
-1.274776551 |
11.41848413 |
Ski11s |
1.640070759 |
4.722713632 |
0.347272964 |
0.730465886 |
-7.947559343 |
11.22770086 |
-7.947559343 |
11.22770086 |
No of Cis |
-0.414975038 |
1.044780866 |
-0.397188589 |
0.693640264 |
-2.535995549 |
1.706045473 |
-2.535995549 |
1.706045473 |
App1ication Fa2i1iarity |
-6.004168977 |
5.308765236 |
-1.130991617 |
0.2657491 |
-16.78154854 |
4.773210585 |
-16.78154854 |
4.773210585 |
Dependency |
3.837841414 |
5.37496051 |
0.714022253 |
0.479948377 |
-7.073921864 |
14.74960469 |
-7.073921864 |
14.74960469 |
Clarification |
7.151995749 |
4.673418171 |
1.530356473 |
0.134917682 |
-2.335559124 |
16.63955062 |
-2.335559124 |
16.63955062 |
Conclusion
Data mining has emerged as an effective tool for businesses across various industries. Extracting valuable insights and patterns from large datasets enables business analysts to make informed decisions, optimize processes, and drive growth.
Through the history of data mining, we can see how this practice has evolved over time, becoming more sophisticated and accessible with advancements in technology. Data mining has come a long way, from its roots in statistics and machine learning to the development of powerful algorithms and tools.
For business analysts, data mining provides a wealth of benefits. It allows them to uncover hidden trends and patterns that may not be apparent through traditional analysis methods. This knowledge equips them with the ability to make accurate predictions about customer behavior, market trends, and potential risks or opportunities.
The process of data mining involves several steps - from understanding the problem at hand and collecting relevant data to cleaning and pre-processing it before applying various techniques like clustering or classification. Each step is crucial in ensuring accurate results that businesses can effectively utilize.
One significant advantage of data mining is its ability to enhance decision-making processes. By providing actionable insights based on historical data analysis, companies can minimize risks, identify cost-saving opportunities, improve efficiency levels, personalize marketing campaigns, and detect frauds or anomalies in real-time operations.
However useful it may be, though; there are also certain limitations associated with data mining. Issues such as privacy concerns related to accessing personal information need careful handling to maintain ethical standards while using customers' private details during analysis procedures.
All things considered, data mining remains an indispensable tool for modern-day business analytics.
The power lies within the hands of those who know how best to harness their collected big raw data sets into intelligent insight-driven actions, which should help organizations stay competitive in today’s fast-paced world.
So whether you're operating a retail store, trying to understand consumer preferences, or analyzing financial markets for investment strategies, data mining offers endless possibilities.
It empowers your organization by transforming complex raw datasets into meaningful insights that ultimately drive success and growth.
You May Also Like
These Related Stories
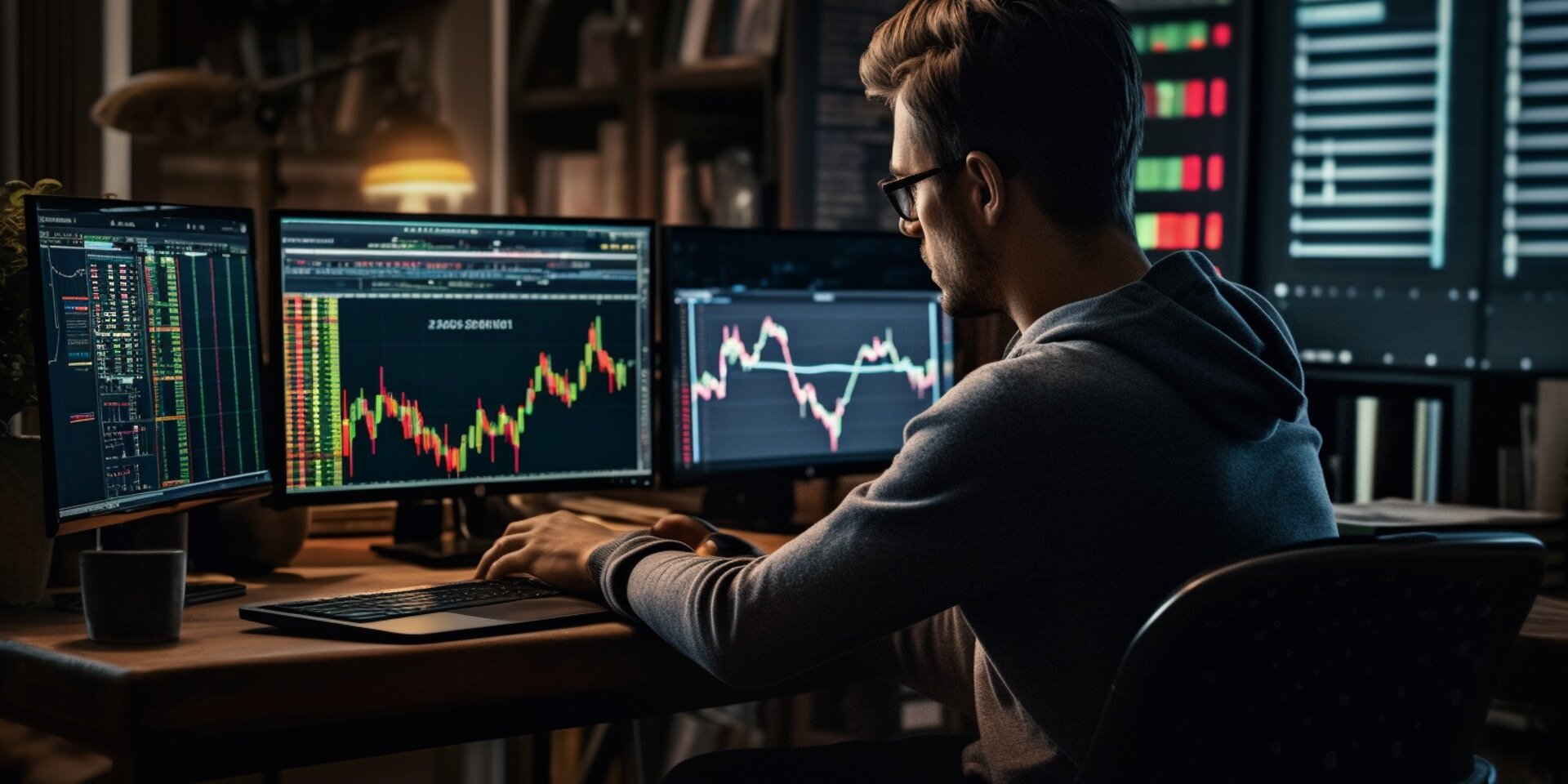
Financial Analysis : Everything That BAs Must Know
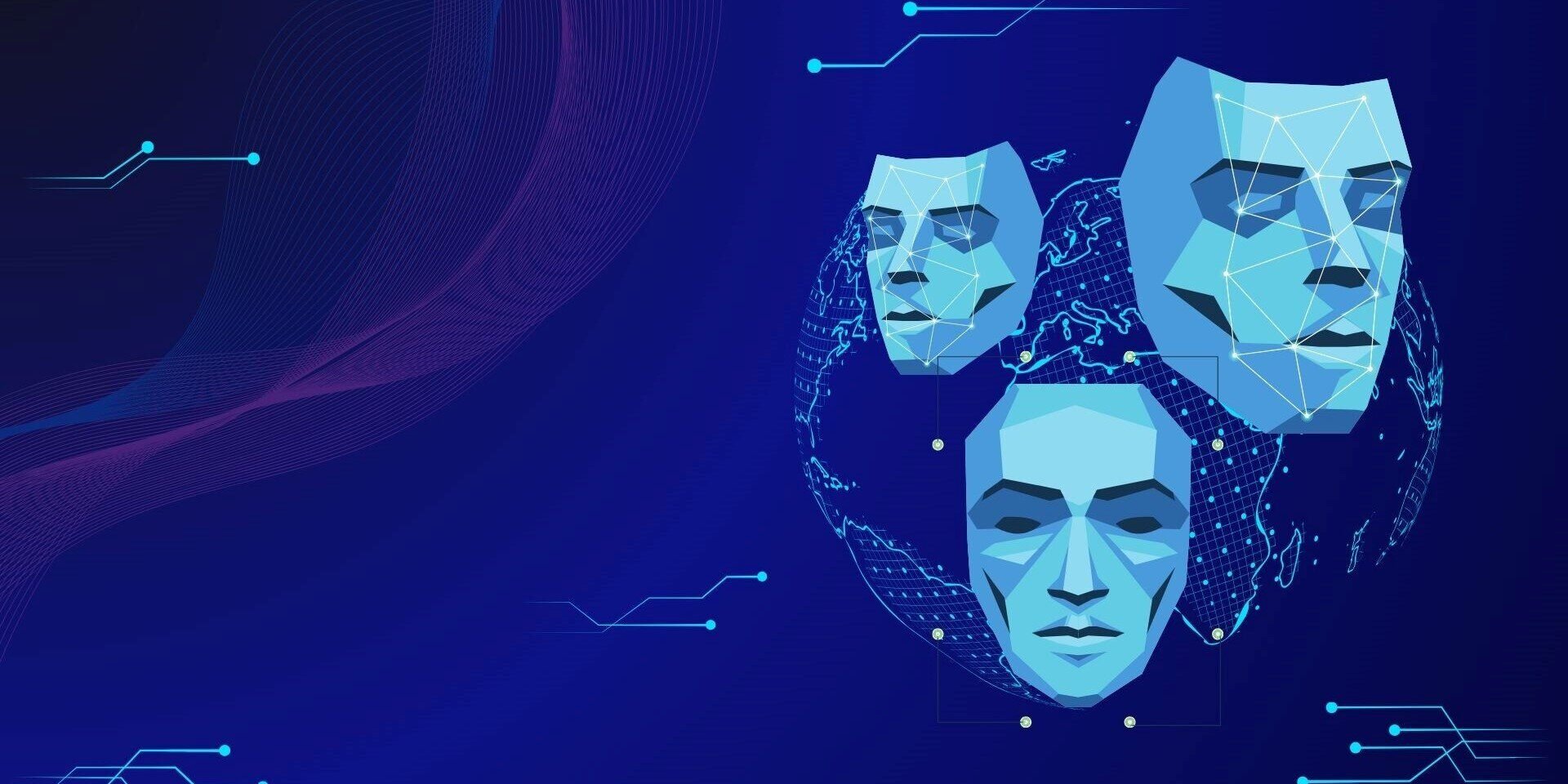
User Persona: Why, How, Examples, and Free Template
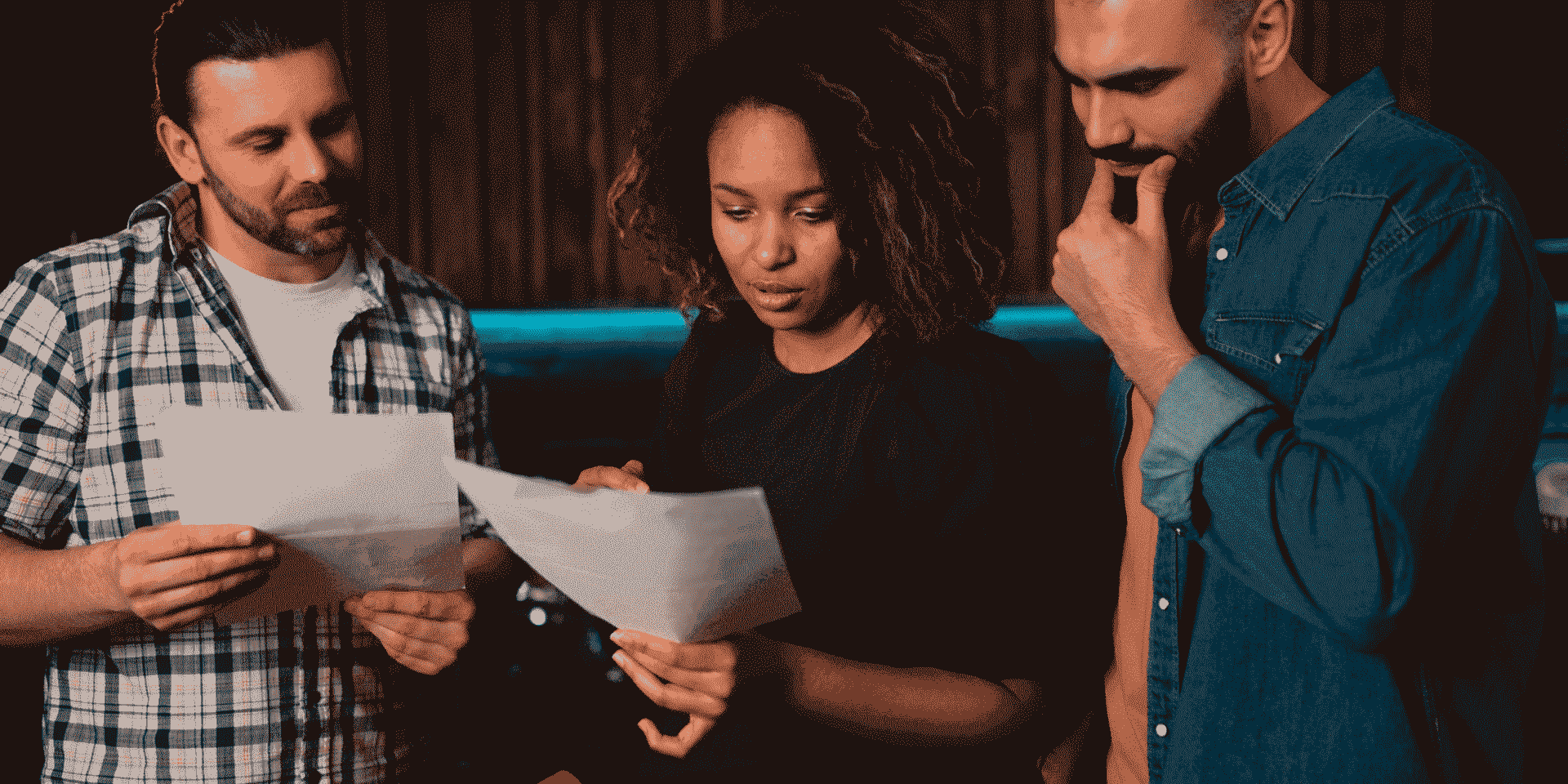
No Comments Yet
Let us know what you think